No More Mixed Signals: How to Listen to Your Buyers, the Right Way
Speakers
Additional resources
No More Mixed Signals: How to Listen to Your Buyers, the Right Way
You often hear dating invoked as a metaphor for the B2B sales cycle—and with good reason. Both are a long process of getting to know each other and determining whether there’s mutual compatibility, before (hopefully) a happily-ever-after ride into the sunset.
If great marketing is like falling in love, you could just as easily compare bad marketing experiences to our modern app-fueled dating hellscape. Consider some common dating complaints—creepy messages, love bombing behavior, or getting ghosted. It’s not all that different from creepy target ads, overzealous outreach, or waiting for a company to (finally) respond to your earnest message.
This leaves marketers in a bind: Come on too strong, and you’ll scare your prospective buyers away. But don’t show enough interest, and your prospective buyers will move on to a competitor who wears their heart on their sleeve. It’s a difficult balance to strike and—like dating—it’s all about picking up your buyers’ signals and responding in a way that makes sense for that phase of the relationship.
So what’s the secret to understanding their signals? As seasoned demand gen experts, we’ve tried all the approaches: lead-scoring, intent data—you name it, we’ve probably done it. But we’ve also found every scoring model lacking in some way. Maybe they rely too much on a buyer taking action, like filling out a form, which only about three percent ever do. Or, maybe they show you which buyers are searching for a product like yours but don’t tell you anything about whether they’re a good fit, leaving you with a ton of noise to sift through.
We knew there had to be a better way. So we did some research. In this article, we’re sharing our findings.
The best of intentions: How we got intent data wrong
Hold up, you might be thinking, I thought we already solved this problem. Isn’t that what intent data is for? To show you who’s in the market for a solution?
But speak to any CMO and you’ll quickly learn that third-party intent data has not lived up to its own hype. A few years ago, everyone was sure that this was the magic solution. Now, we hear bitter marketers complaining that it isn’t actually deterministic to winning deals—all it gives them is noise.
Maybe the problem is we were all looking at it with rose-tinted glasses. Because the truth is, third-party intent data was never intended as your only source of buyer information. It’s only one piece of the puzzle. When teams rely on it exclusively instead of looking holistically at their buyers’ behavior online, they set themselves up for disappointment. It’s like assuming everyone who likes your dating profile is worth going on a date with—you need more information before you can decide whether it’s worth asking them to grab drinks.
That’s not to say that you shouldn’t use third-party data, but just that you need to understand where it fits and what insights it can—and can’t—provide. So what does that mean about how you should use third-party intent data? And how do you gain a deeper understanding of your prospective customers? We’ll tackle that in our next section.
How to read your customers’ signals
You might have noticed we’ve been using the term “signals” in this article instead of “intent data.” That’s on purpose. “Signals” refers to all your customer data sources—not just the third-party data you’d get from an intent data provider. It encompasses the first-party data on your website, the second-party data you get from review sites, plus third-party data sources.
The secret to getting a clear picture of how your customers are thinking and feeling? Mixing and matching different types of signal data so you can get a better view. There’s a hierarchy here: First-party data is typically the best quality, then second-party data, then third. This is inversely related to how easy they are to get: anyone can buy third, you need good partnerships to get second (or you can purchase it), and first requires real work to set up.
While first-party data is always going to be the most reliable, there can be a finer line between second- and third-party data. Second-party data should theoretically be more reliable, but if you’re drawing from multiple third-party sources and just one second-party data source, the third-party data may actually be giving you a more complete picture. It all depends on how you’re mixing and matching your data.
Here’s a high-level overview of the different sources you can consider drawing from:
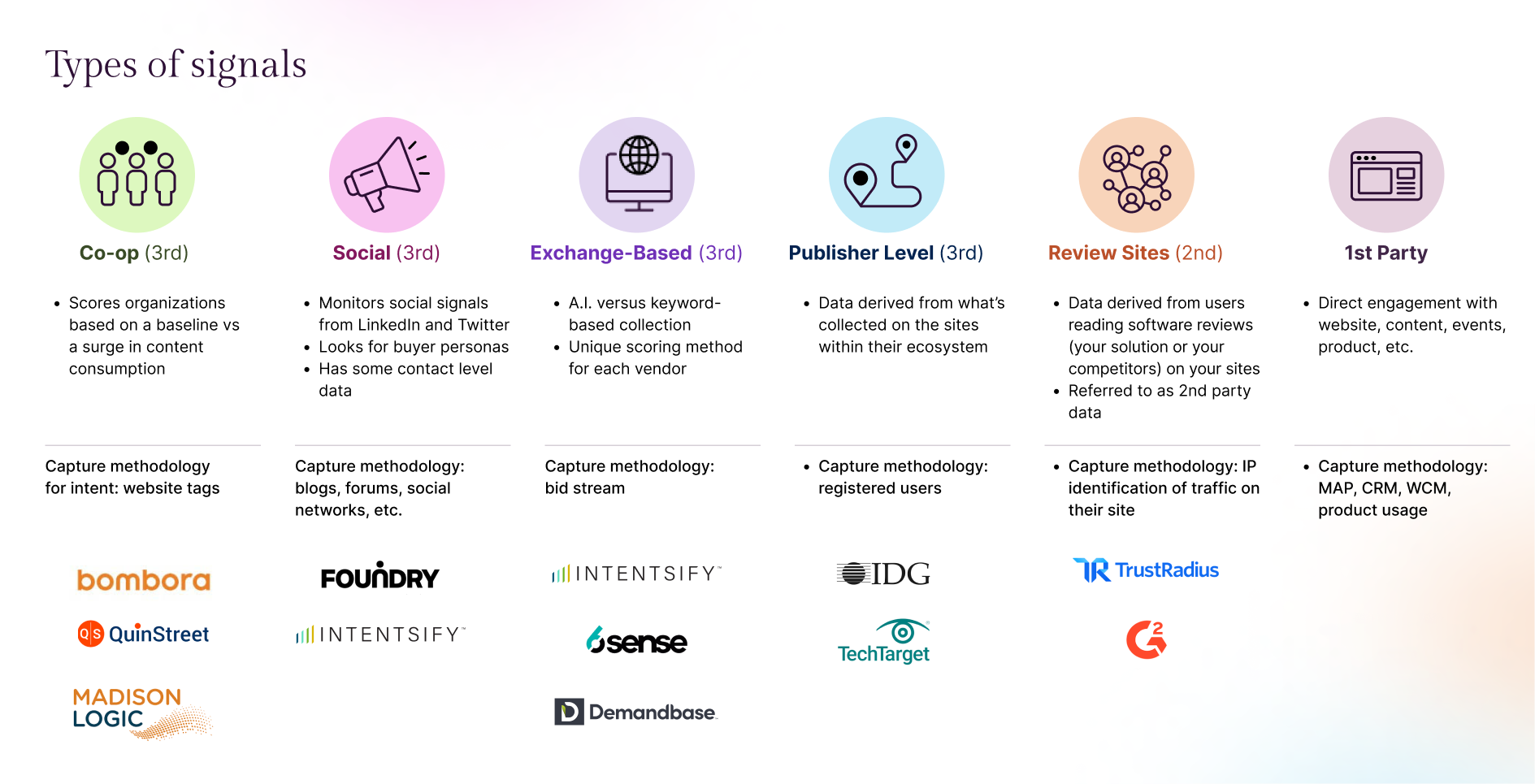
You might not be leveraging all of these different sources of data, and that’s okay. This is just a high-level overview of what you could be using. Start by nailing down your higher-quality data sources (first- and second-party), then round it out with third-party data to give you a more holistic view.
The other missing piece: Figuring out who you want to attract
Just layering these different sources of data isn’t enough to give you a good score. It’s still missing one critical piece: how well these buyers match your ideal customer profile (ICP). If you’re on the dating apps looking for someone to settle down with in a quaint countryside cottage so you can fulfill your lifelong dream of raising chickens, you’re probably not a great match for a high-powered corporate type who wants to live in the big city—no matter how much they might like you. Similarly, a good score is one that rationalizes how interested you are in a buyer (ICP fit) with how interested they are in you.
A good score is one that rationalizes how interested you are in a buyer (ICP fit) with how interested they are in you.
A common marketing mistake is trying to appeal to a wide audience instead of understanding who fits your solution best. Understanding your best-fit customers allows you to focus your efforts on the buyers who are going to be great-fit, long-term customers—not the ones who will churn next quarter when they realize your tool doesn’t quite fit with what they were trying to accomplish or they can no longer justify the cost.
That’s why we recommend first creating an ICP fit score. You can do this by assigning points to certain qualities that your ideal buyers tend to have and weighting them to create an overall score. To create this scoring system, you can consider:
- Firmographics: Business information like industry, company size, annual revenue, geography, number of employees, and so on.
- Technographics: Specific information around technology usage such as tech stack, cloud services, IT infrastructure, security, and preferences around software.
- Other relevant information: Things like whether the potential buyer is already a client of one of your ecosystem partners, relevant spend to your offering, the lifetime value (LTV) of your existing customers (and their characteristics), and other details that may be specifically relevant to your business.
Once you have a way of measuring your potential buyers’ fit—and only then—you can start to factor in the signals they’re giving you that they’re interested in making a purchase.
So how do you weigh ICP fit along with your data sources in order to come up with a score that’s meaningful? We’ll tackle that next.
Bringing it all together: How to determine whether it’s a match
Meet the ICP vs. signals matrix. This is how we at Inverta rationalize ICP fit with a buyer’s propensity to convert.

On the x-axis you have your buyer’s interest (what you know based on the signals you collect), while on the y-axis you have the buyer’s ICP fit score (how good a match they are for your solution). Your best potential customers are in the upper right section—high ICP fit, high interest in you.
Rationalizing your signals so you can factor them into this combined score is a lot trickier because you need to aggregate a lot of different sources of data across all the members of the buying committee (today, there are an average of eleven people involved). “Think of it like lead-scoring to the third power,” says Kathy Macchi, co-founder of Inverta. If earlier versions of lead-scoring were two-dimensional, signals-based marketing is a 3D cube, because you’re taking into consideration all of those different buyers and the interactions they’re having with your business.
Adding to the challenge, most of today’s martech tools aren’t set up for this kind of signal aggregation—they’re set up for traditional lead scoring. That means companies need to find their own individual workarounds. For instance, you can use a tool like Zapier to combine, normalize, weight/rank, rationalize, and interpret all the signals into an actionable score or data point, then push it to your MAP or CRM to action on it, as illustrated in the flowchart below.

Of course, some of the old guidance still holds true: Like with lead-scoring, you’re going to need to weight your data sources (e.g. first-party data gets more weight than third-party data) and set it up so your data degrades over time, so recent interactions take precedence over old ones. You’re just juggling a lot more different sources of data now.
It’s important to note that this isn’t going to be an overnight process—if your entire marketing infrastructure is set up around MQLs, you can’t change to be signals-based tomorrow. It’s a slow, iterative process. But the more you can do to take your buyers’ signals into consideration, the better off you’ll be in the long run. “We're all figuring it out right now, but buyers have changed how they're buying and our infrastructure is aligned to how they used to buy,” says Kathy. “Whoever can figure this out sooner is going to be in better shape.”
Getting to signals-based marketing
Like dating, the B2B buying journey can be tough for both sides. But it is still possible to find “true love”—you just need to be savvy and smart about it. Don’t rely exclusively on third-hand information. Prioritize first-party interactions and high-quality data. Don’t try to appeal to everyone. Make a list of what you’re looking for, filter heavily for those qualities, and pay careful attention to the signals your buyers are sending so you can spot the green and red flags. And leave a little margin for error—all of our friends who found partners on dating apps ended up being at least a bit surprised. (Marketers too, leave some room for hand-raisers and don’t assume the model is everything.)
If you’ve been an attentive reader, you might recognize that this article is also about change management. We’ve all been focused on MQLs for a long time and it’s not possible to tear out all that infrastructure and start fresh overnight—as much as we might wish it were. “I see it as the same as when we went from product selling to solution selling,” says Kathy. “That was a two-year process, if not three.” There’s going to be moments of awkwardness and mixed signals as we adapt. That’s inevitable. But as our buyers change, we need to grow and change alongside them. Otherwise, we’ll miss more than signals—we’ll miss out on opportunities and revenue.